Intro
I, like tens of millions around the globe, have played the EA FIFA game series for more than two decades. But beyond its entertainment capabilities, it possesses a vast dataset of full player ratings, market value and metadata spanning over a decade, granting a great opportunity to learn about the development of football players over time.
The FIFA project is my 2nd series that meets data science with the world's most beloved sport. This mini-series will focus on what football insights we can squeeze out of the EA FIFA dataset alone.
Starting from the basics, and gradually tackling more complex (and useful) tasks that require heavier mathematical tools. Ultimately, the voyage ends
-
Chapter 1: What Comes and Goes with Age
-
Chapter 2: Player Valuation
-
Chapter 3: Predicting the Future
#1 What Comes and Goes with Age
Delving into the intricate relationship between age and football performance, covering the questions:
How on- and off-ball skills, evolve with age
Why attackers and defenders affected differently
Golden age or a tipping point? Why the ages of 23-24 are crucial in players careers?
Examining nations and league based trends
Introducing Career phases - a robust clustering-based approach to explore and understand players development





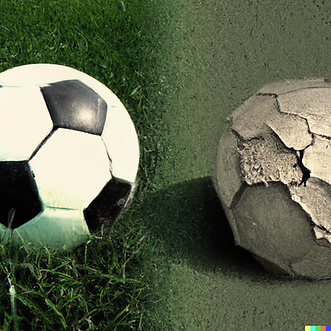
Footballers evolution
Career phases

Acc-decay
Decrease of ≥ 4 points in overall rating YoY
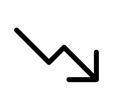
Mid-decay
Decrease of 1-3 points in overall rating YoY
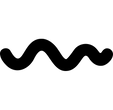
Stagnation
Between -1 to +1 points change in overall rating YoY

Mid-growth
Increase of 2-3 points in overall rating YoY
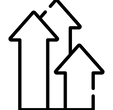
Acc-growth
Increase of ≥ 4 points in overall rating YoY
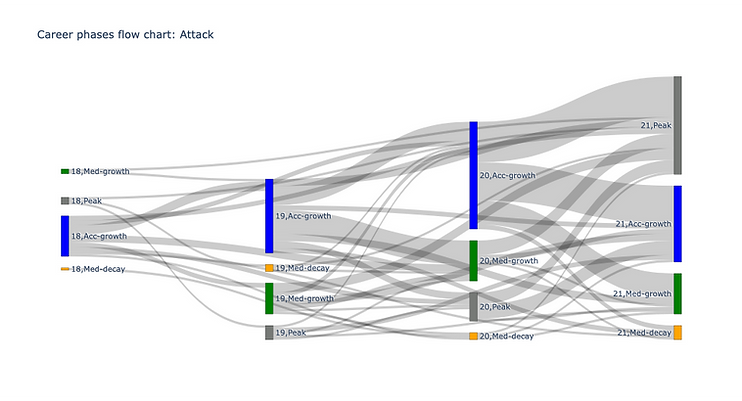
The career phases graph for attackers between the ages of 18–21. Each node is permutation of (age, career phase). The height of a node’s rectangle reflects its sample size, color by career phase.
The Tale of D. Alli and O. Watkins
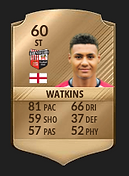

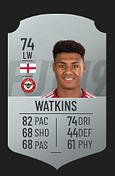
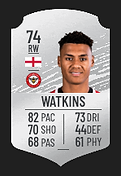
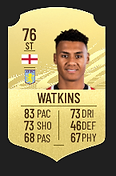

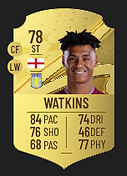
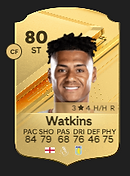
2017 2018 2019 2020 2021 2022 2023 2024
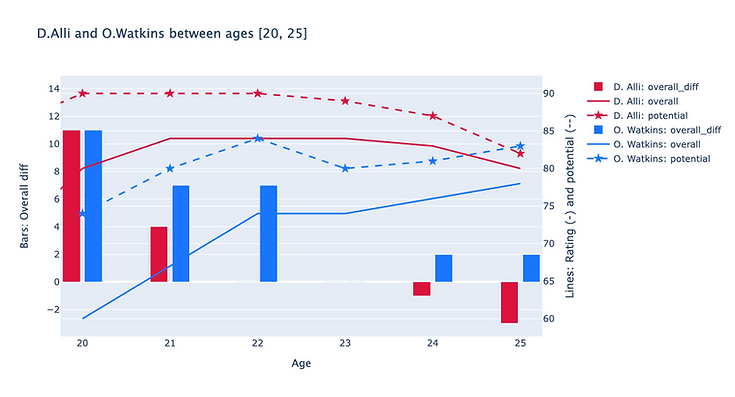
#2 Player Valuation
This section is a work in progres...
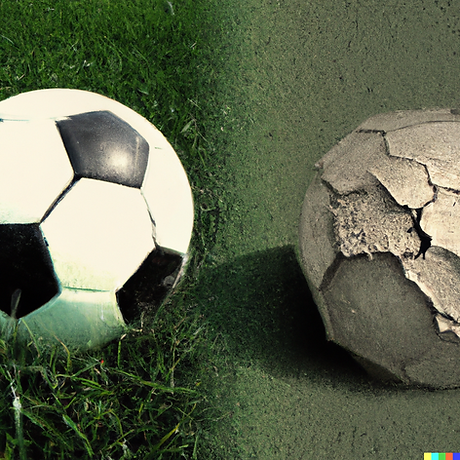